M.Sc. Daniel Plabst
Technical University of Munich
Chair of Communications Engineering (Prof. Kramer)
Postal address
Postal:
Theresienstr. 90
80333 München
Biography
I received my M.Sc. degree from the Technical University of Munich (TUM) in 2018.
In my Master's thesis I worked on signal processing for energy-efficient wireless communication systems at the Associate Professorship of Signal Processing (MSV), while staying with the Wireless Networking and Communications Group at the University of Texas at Austin (UT), USA.
Since 2019, I have been working as a research associate at the Institute of Communications Engineering. From 2019 to 2022 I was supervised by Prof. Norbert Hanik. Since 2023 I am supervised by Prof. Gerhard Kramer.
Research Interests
Direct-Detection (DD) is a cost-effective approach for short-reach fiber-optic communication. A DD receiver uses a single photodiode to measure the intensity of the impinging signal. This suggests that DD is not compatible with phase modulation. However, using oversampling, the phase can be recovered by exploiting inter-symbol interference (ISI).
We investigate DD for short-reach communication in the context of information theory and estimation strategies.
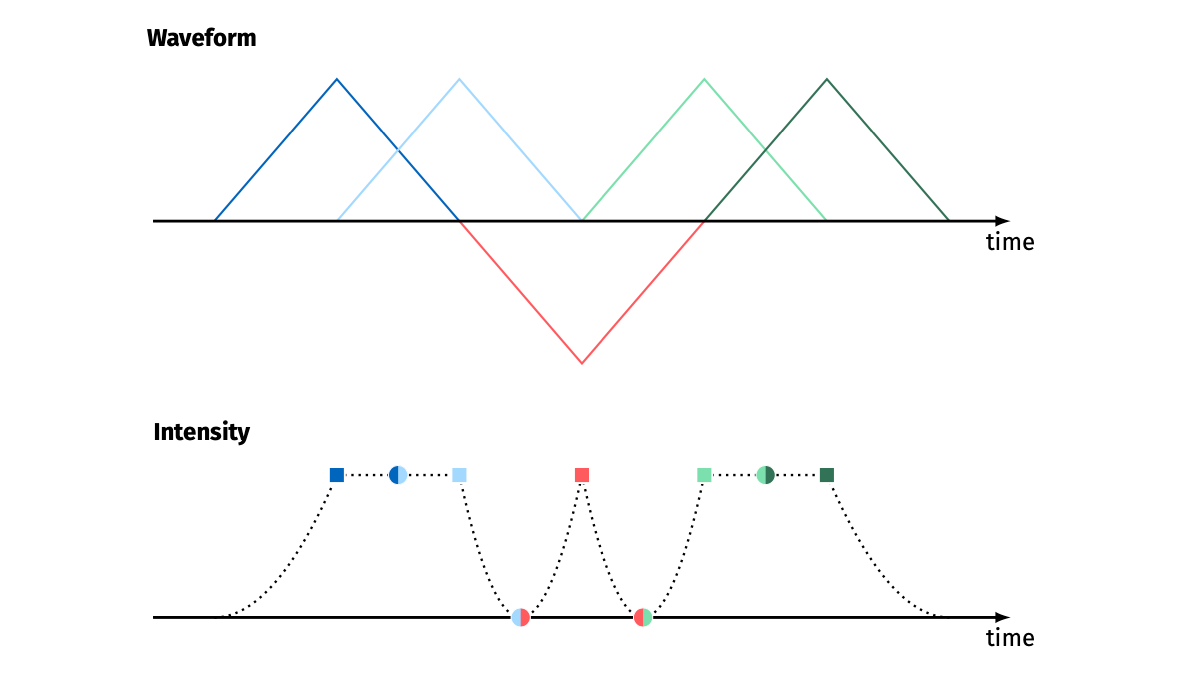
Teaching
Optical Communication Systems (ST 2019, WT 2020)
Digital Signal Processing for Optical Communication Systems (ST 2020, 2021)
Physical Layer Methods (ST 2023)
Basic Laboratory Course on Telecommunications (ST 2022, WT 2022, ST 2023, WT2023)
Communications Laboratory (WT 2022, WT2023)
Nachrichtentechnik 2 (WT 2023)
Theses in Progress
Achievable Rates for a Constrained Gaussian Channel
Description
Input-constrained Gaussian waveform channels are considered in [1]. The channel consists of a linear filter and Gaussian noise is added at the receiver.
The transmitter generates a waveform x(t). While x(t) has no bandwidth limit, the authors compare the channel capacity under
- a value constraint on x(t) such that x(t) can take only two values,
- and a peak-power constraint.
The paper shows that capacity under both constraints is equal.
Input-constrained channels with a value constraint are of great importance for applications such as magnetic storage media.
The student should understand and be able to interpret why capacity is equal under constraints 1. and 2., and how according lower bounds are obtained.
[1] L. H. Ozarow, A. D. Wyner and J. Ziv, "Achievable rates for a constrained Gaussian channel," in IEEE Transactions on Information Theory, vol. 34, no. 3, pp. 365-370, May 1988, doi: 10.1109/18.6018.
Prerequisites
- Information theory.
- Linear Systems Theory.
Supervisor:
Neural Network-Based Signal Predistortion for Direct Detection Systems
Description
During the internship, the student will be researching the application of Neural Network-based signal predistortion to mitigate the effects of fiber chromatic dispersion in direct detection systems.
Prerequisites
- basic Python skills beneficial
Supervisor:
Rate upper Bounds for Bandlimited Channels with a Memoryless Nonlinearity
Description
We are interested in computing upper bounds (on capacity) for bandlimited channels with a memoryless nonlineary at the transmitter/receiver.
One application for these bounds are short-reach fiber-optic communication systems with a single photodiode at the receiver. The photodiode is a memoryless nonlinearity, as it produces an output that is proportional to the squared magnitude of the input signal.
A simple upper bound for the above model is given in [Sec. III D, 2].
D. Plabst et al., "Achievable Rates for Short-Reach Fiber-Optic Channels With Direct Detection," in Journal of Lightwave Technology, vol. 40, no. 12, pp. 3602-3613, 15 June15, 2022, doi: 10.1109/JLT.2022.3149574.
Prerequisites
Information Theory
Linear System Theory
Supervisor:
2024
- Neural network equalizers and successive interference cancellation for bandlimited channels with a nonlinearity. IEEE International Symposium on Information Theory, 2024 more… BibTeX
- Neural network equalizers and successive interference cancellation for bandlimited channels with a nonlinearity. IEEE Trans. Commun., 2024 more… BibTeX
- Neural Network Equalizers and Successive Interference Cancellation for Bandlimited Channels with a Nonlinearity. arXiv, 2024 more… BibTeX
2023
- Neural Network Successive Interference Cancellation for Channels with Intersymbol Interference and a Memoryless Nonlinearity. ITG Professional Group "Applied Information Theory", 2023Stuttgart more… BibTeX
- Successive Interference Cancellation for Fiber-Optic Channels with Direct Detection. ITG-Fachgruppe KT3.1, 2023München more… BibTeX
- Successive Interference Cancellation for Bandlimited Channels with Direct Detection. IEEE Trans. Commun., 2023 more… BibTeX
2022
- Achievable Rates for Short-Reach Fiber-Optic Channels with Direct Detection. IEEE/OSA J. Lightw. Technol., 2022 more… BibTeX Full text ( DOI )
- Comparison of PAM-6 Modulations for Short-Reach Fiber-Optic Links with Intensity Modulation and Direct Detection. European Conference on Optical Communication (ECOC) 2022, 2022 more… BibTeX Full text ( DOI )
- Experiments on Bipolar Transmission with Direct Detection. European Conference on Optical Communication (ECOC) 2022, 2022 more… BibTeX Full text ( DOI )
2021
- Sparse Linear Precoders for Mitigating Nonlinearities in Massive MIMO. IEEE Statistical Signal Processing Workshop 2021, 2021 more… BibTeX
- PAM-6 Coded Modulation for IM/DD Channels with a Peak-Power Constraint. International Symposium on Topics in Coding (ISTC) 2021, 2021 more… BibTeX
- Recovering the Phase in DD Receivers with Oversampling: A Toy Example. Huawei Joint Lab Workshop, 2021 more… BibTeX
2020
- Back-to-Back Performance of the Full Spectrum Nonlinear Fourier Transform and Its Inverse. Entropy 22 (10), 2020, 1131 more… BibTeX Full text ( DOI ) Full text (mediaTUM)
- Stability of the Full Spectrum Nonlinear FourierTransform. International Conference on Transparent Optical Networks (ICTON) 2020, 2020 more… BibTeX Full text (mediaTUM)
- Phase-Retrieval for Short-Reach IM/DD Links. Workshop der Informationstechnischen Gesellschaft (ITG) in Karlsruhe, 2020 more… BibTeX
- Wiener Filter for Short-Reach Fiber-Optic Links. IEEE Communications Letters 24 (11), 2020, 2546 - 2550 more… BibTeX Full text ( DOI )
2019
- Linear Transmit Signal Processing in 1-Bit Quantized Massive MIMO Systems. 2019 Munich Doctoral Seminar on Communications, 2019 more… BibTeX Full text (mediaTUM)
2018
- Efficient Equalization Method for Cyclic Prefix-Free Coarsely Quantized Massive MIMO Systems. 2018 IEEE International Conference on Communications (ICC), 2018 more… BibTeX Full text ( DOI )
- Efficient Non-linear Equalization for 1-bit Quantized Cyclic Prefix-Free Massive MIMO Systems. 2018 15th International Symposium on Wireless Communication Systems (ISWCS), 2018 more… BibTeX Full text ( DOI )