- Composition Counts: A Machine Learning View on Immunothrombosis using Quantitative Phase Imaging. Machine Learning for Healthcare Conference, 2023 more… BibTeX
- Towards Interpretable Classification of Leukocytes based on Deep Learning. 3rd Workshop on Interpretable Machine Learning in Healthcare (IMLH), 2023 more… BibTeX
- Explainable Artificial Intelligence for Cytological Image Analysis. In: Artificial Intelligence in Medicine. Springer Nature Switzerland, 2023 more… BibTeX Full text ( DOI )
- Rethinking Usability Heuristics for Modern Biomedical Interfaces. The Sixteenth International Conference on Advances in Computer-Human Interactions (ACHI), 2023 more… BibTeX
- Measurement of Platelet Aggregation in Ageing Samples and After in-Vitro Activation. Proceedings of the 16th International Joint Conference on Biomedical Engineering Systems and Technologies, SCITEPRESS - Science and Technology Publications, 2023 more… BibTeX Full text ( DOI )
- Explainable Feature Learning with Variational Autoencoders for Holographic Image Analysis. Proceedings of the 16th International Joint Conference on Biomedical Engineering Systems and Technologies, SCITEPRESS - Science and Technology Publications, 2023 more… BibTeX Full text ( DOI )
- Label-free Digital Holographic Microscopy to Characterize Inter- and Intratumoral Heterogeneity in Pancreatic Cancer. Gastroenterology 162 (7, Supplement ), 2022, S-732 more… BibTeX Full text ( DOI ) WWW
- Blutzellaggregate als neue Biomarkerklasse für POC Hämatologie Automaten. 5. MÜNCHNER POCT SYMPOSIUM, Neue Perspektiven für Querschnittstechnologien und erweiterte Anwendungsgebiete , 2022, 68 more… BibTeX
- A Comparison of Uncertainty Quantification Methods for Active Learning in Image Classification. 2022 International Joint Conference on Neural Networks (IJCNN), IEEE, 2022 more… BibTeX Full text ( DOI )
- Outlier Detection using Self-Organizing Maps for Automated Blood Cell Analysis. Interpretable Machine Learning in Healthcare Workshop, ICML 2022, 2022 more… BibTeXWWW
CellFace
Establishing digital, holographic flow cytometry as a label-free platform technology for cellular diagnostics.
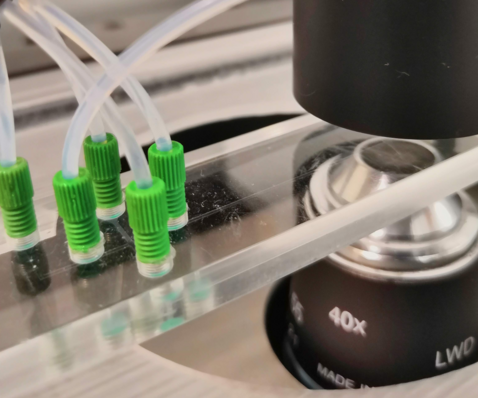
CellFace aims to establish digital, holographic flow cytometry as a label-free platform technology for cellular diagnostics. We are an interdisciplinary project of the Technical University of Munich, coordinated by the Chair of Biomedical Electronics and the Chair of Data Processing in cooperation with several departments of the University Hospital rechts der Isar, the Professorship for Methods of Signal Processing and the Chair of Integrated Systems.
We are developing a novel in vitro diagnostic method for cellular analysis and biomarkers that are not currently accessible for clinical routine. With access to clinical samples, a highly accurate sample presentation and an imaging solution, we can offer AI-driven data exploration and interpretation of volatile biomarker panels.
A tiny drop of blood gives us a detailed picture of a patient's health and allows us to support clinicians in their decision making. Our label-free technology enables us to provide insights into various aspects of health, ranging from cardiovascular risk assessment to leukemia detection, all within minutes.
For further information refer to the CellFace Wiki.
Use Cases
Hematology:
Blood provides many insights into the health of an organism, especially in the field of in vitro diagnostics. As a result, hematological analyses such as complete blood count (CBC) represent a large proportion of laboratory tests in the healthcare sector. Despite the availability of largely automated analyzers, the gold standard for routine diagnosis of hematological disorders is the laborious Giemsa-stained blood smear, which suffers from inter-observer variability. Other drawbacks of current approaches include high sample preparation effort, material cost, long processing time, or lack of clinical relevance. The CellFace project was initiated to address these issues and in particular to reduce time-consuming manual intervention. Flow cytometry combined with quantitative phase imaging allows researchers to capture 3D images of each individual cell while maintaining a high-throughput flow of blood cells. The overall goal is to establish this label- and reagent-free approach as a platform technology for automated blood cell diagnostics. The best methods to analyze the recorded phase images are still under investigation. Therefore, classical and modern techniques in the field of computer vision and machine learning will be investigated for their suitability to interpret the images and the information they contain.
Researchers
PIs
Scientific Staff
- Christian Klenk
- Dominik Heim
- Stefan Röhrl
- Manuel Lengl
- Simon Schumann
- Martin Knopp
- Ellen Emken
- Julia Sistermanns