Neuroengineering
Contact: Nicolas Berberich
Reference: Gordon Cheng, Stefan K. Ehrlich, Mikhail Lebedev and Miguel A. L. Nicolelis: Neuroengineering challenges of fusing robotics and neuroscience. Science Robotics (Vol. 5, Issue 49, eabd1911), 2020. DOI: 10.1126/scirobotics.abd1911
Neuroengineering is an emerging research field which is characterized by the synergistic combination of theories and methods from neuroscience and engineering.
In one direction, methods from engineering such as signal processing, control and information theory, machine learning, electronic recording and stimulation interfaces, robotic manipulanda and neural imaging techniques are used to study the central scientific questions of neuroscience and cognitive science: “How does the brain work? How does it produce complex, goal-oriented and adaptive behavior and associated mental phenomena?”
In the other direction, concepts, theories, and methods from neuroscience are employed to build better technical system. Here, two distinct research themes can be distinguished. One research theme – Neuro-inspired Technical Systems - takes inspiration from neural principles in order to improve the efficiency and robustness of engineering systems. Examples for this are neuromorphic systems such as dynamic vision sensors, neuromorphic chips, and our robot skin, which employ concepts from neuroscience, e.g., event-based communication and sparse coding to achieve significantly lower energy consumption than traditional engineering approaches. Another highly valuable perspective that neuroscience adds to engineering and especially to AI research is the emphasis on the embodiment of cognition and its dependency on sensorimotor and social interactions. As a consequence, embodied cognitive systems such as humanoid robots can be considered as a powerful research platform for studying human cognition as well as for pushing the frontier of engineering.
The second research theme which applies neuroscientific knowledge towards building better technical systems is Brain-Machine Interface (BMI) research, which studies the interfaces, interactions and co-adaptivity between nervous systems and machines such as robots, computers, neuroprostheses and exoskeletons.
Neuro-ergonomic human-robot interaction (HRI): Error-related potentials based passive brain-computer interfaces for HRI
Contact:
Abstract: Successful collaboration requires interaction partners to constantly monitor and predict each other’s behavior to establish shared representations of goals and intentions (Tomasello & Carpenter, 2007). In human-robot interaction (HRI) this aspect is particularly challenged by ongoing technical limitations in robot perception and reasoning about the human partner’s behavior and underlying intentions (Hayes & Scassellati, 2013). The human partner on the other hand is not just capable of judging the robot behavior but also to oversee the overall interaction performance. Studies have demonstrated that erroneous or unexpected robot actions engage error-/performance processes in the human partner’s brain, which manifest as error-related potentials (ErrPs), observable and reliably decodeable using non-invasive electroencephalography (EEG) (Ehrlich & Cheng, 2016). Real-time decoded ErrPs constitute a valuable source of information about the human partner’s expectations and subjective preferences (Botvinick et al., 2004) and therefore could serve as a useful complement to existing methods for validating and improving HRI or human-machine interaction in general (Kim et al., 2017). The central question of this research is to develop methods to harvest and utilize these ErrPs for validation or adaption of robot behavior in HRI. A particular focus lies on investigating the usability of ErrPs for mediating co-adaptation in social interactive and collaborative HRI where robots appear as intentional agents and mutual adaptation between human and robot is required (Ehrlich & Cheng, 2018).
References:
Tomasello, M., & Carpenter, M. (2007). Shared intentionality. Developmental science, 10(1), 121-125.
Hayes, B., & Scassellati, B. (2013). Challenges in shared-environment human-robot collaboration. learning, 8(9).
Botvinick, M. M., Cohen, J. D., & Carter, C. S. (2004). Conflict monitoring and anterior cingulate cortex: an update. Trends in cognitive sciences, 8(12), 539-546.
Kim, S. K., Kirchner, E. A., Stefes, A., & Kirchner, F. (2017). Intrinsic interactive reinforcement learning–Using error-related potentials for real world human-robot interaction. Scientific reports, 7(1), 17562.
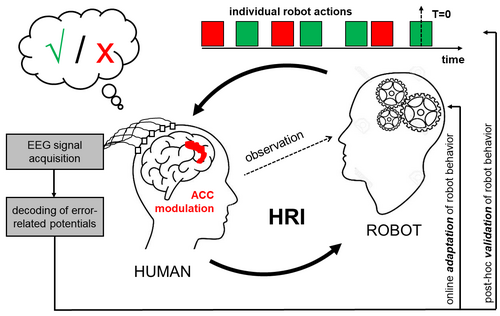
Brain Sound Computer Interface
Decoding sound sequences from human brain by machine learning techniques
Contact: Alireza Malekmohammadi
Description: When we hear a melody, music, or any other sequential sounds frequently, we could memorize it in way that it is possible to anticipate the rest of music by hearing the first part of that. It means the music or melody is encoded/stored inside of the brain in such a way that we could imagine/retrieve these sequences of sounds. Although considerable achievement has been done during the last decade in understanding of processing complex sounds by brain, the coding and storage of sequential tones are sill poorly understood (Rauschecker, Josef P, 2005). One of the unsolved challenging in understanding human brain particularly auditory neuroscience is how brain encodes sequences of sounds and stores them (Rauschecker, Josef P, 2011), and How we could decode brain regarding these stored sounds. Sequences of sound could be familiar or unfamiliar to human auditory part of brain. In other words, we are planning to address this question that how brain neurons interacts with each other regarding both familiar and unfamiliar continuous auditory stimulus.

Brain-Machine Interface (BMI)
Contact: Nicolas Berberich
BMI research studies how humans can non-verbally communicate their intentions to robotic devices through their neural activity. The neural activity is measured with technical devices such as EEG or EMG recording systems and interpreted through advanced signal processing and machine learning methods. Additionally, other neural information such as cognitive load and whether the human has recognized an error can be recognized to improve the quality and convenience of the human-machine interaction. Besides this efferent pathway from the human to the machine, the information flow from the machine to the human through sensory feedback or substitution or through neural stimulation is also an essential part of BMI research. Brain-machine interfaces in the form of assistive and rehabilitative systems offer a huge potential for neurological patients and disabled people, however because the human is quite literally in-the-loop, as a sensorimotor agent but also as a psychological and social being, a human-centered approach to brain-machine interfaces is of central importance. This includes the involvement of all important stakeholders such as the medical experts and most importantly the patients themselves, as well as a special focus on embedding values in engineering design such as safety and comfort through soft robotic interfaces.
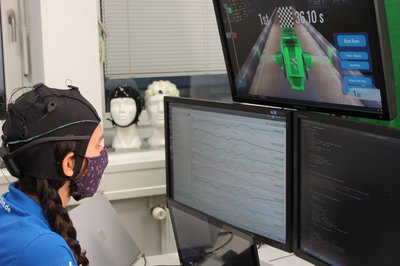
Neurorobotics
The field of neurorobotics studies the interface between robots and biological neural systems. Instead of investigating autonomous robotic systems which are supposed to move and act without input from humans, the neurorobotic approach investigates how purposive robotic behavior can emerge from the combination of a human mental states and intentions with computational methods of sensing and modelling the system's environment. A core focus on the interface between humans and robots lies in the co-adaptation between robots and the human brain and the question of how to build robots whose behavior and control are adapting to the specifics of the brain and neural system, while taking into account that neural plasticity will result in the brain constantly adapting to the robotic system as well.
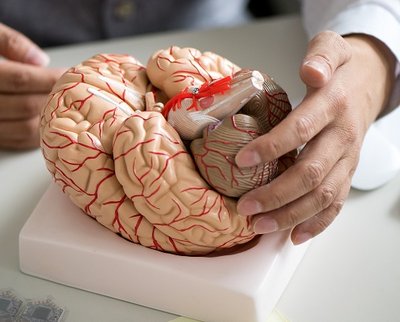