2nd International Workshop on "Data Driven Intelligent Vehicle Applications"
DDIVA 2020
30th October, 2020
A workshop in conjunction with IV 2020 in Las Vegas, NV, United States
Starts at 8 am (Las Vegas Time), 4 pm (Germany Time)
Zoom link: https://tum-conf.zoom.us/j/97355193098?pwd=984458
Password: 984458
Home
Recent advancements in the processing units have improved our ability to construct a variety of archi- tectures for understanding the surroundings of vehicles. Deep learning methods have been developed for geometric and semantic understanding of environments in driving scenarios aim to increase the suc- cess of full-autonomy with the cost of large amount of data.
Recently proposed methods challenge this dependency by pre-processing the data, enhancing, collecting and labeling it intelligently. In addition, the dependency on data can be relieved by generating synthetic data, which alleviates this need with the cost-free annotations, as well as using the test drive data from the sensors and hardware mounted on a vehicle. Nevertheless, state of the driver and passengers inside the cabin have been also of a big importance for the traffic safety and the holistic spatio-temporal perception of the environment.
Aim of this workshop is to form a platform for exchanging ideas and linking the scientific community active in intelligent vehicles domain. This workshop will provide an opportunity to discuss applications and their data-dependent demands for spatio-temporal understanding of the surroundings as well as inside of a vehicle while addressing how the data can be exploited to improve results instead of changing proposed architectures.
Please click to view the last year's workshop DDIVA'19.
Important Dates
Workshop paper submission: March 14th, 2020
Notification of workshop paper acceptance: April 18th, 2020
Final Workshop paper submission: May 2nd, 2020
DDIVA Workshop in 30th October, 2020
Please also check the conference web page for updates.
Preliminary Workshop Program
Start | End | |
---|---|---|
8:00 | 8:10 | Introduction & Welcome |
8:10 | 8:50 | Dominik Notz - Agent and Perception Models for Realistic Simulations |
8:50 | 9:30 | Omesh Tickoo - Robust Visual Scene Understanding under Uncertainty |
9:30 | 9:45 | Break |
9:45 | 10:25 | Eren Erdal Aksoy - Uncertainty-aware Semantic Segmentation of LiDAR Point Clouds for Autonomous Driving |
10:25 | 11:05 | Ekim Yurtsever - Assessing Risk in Driving Videos using Deep Learning |
11:05 | 11:25 | Ralf Graefe - Intel Labs Europe - Providentia ++ Project |
11:25 | 12:00 | Panel Discussion with All Speakers |
12:00 | 12:10 | Closing |
Confirmed Keynote Speakers
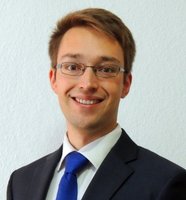
Speaker | Dominik Notz |
Affiliation | BMW Group Research, New Technologies, Innovations - Technology Office USA |
Title of the talk | Agent and Perception Models for Realistic Simulations |
Abstract | Simulation and reprocessing are crucial components for the assessment of automated vehicles. Current methods for simulating and reprocessing driving scenarios lack realistic agent and perception models. The lack of such models introduces a significant source of errors and can render experiment outcomes invalid. We present a methodology to leverage infrastructure sensor recordings from the real world to derive both agent and perception models. Such models need to be scenario- / maneuver-specific. When combined with an approach to automatically extract and cluster driving scenarios, these models can increase simulation realism and validity. |
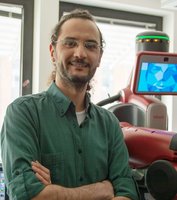
Speaker | Eren Erdal Aksoy |
Affiliation | Halmstad University |
Title of the talk | Uncertainty-aware semantic segmentation of LiDAR point clouds for Autonomous Driving |
Abstract | Scene understanding is an essential prerequisite for autonomous vehicles. Semantic segmentation helps to gain a rich understanding of the scene by predicting a meaningful class label for each individual sensory data point. Safety-critical systems, such as self-driving vehicles, however, require not only highly accurate but also reliable predictions with a consistent measure of uncertainty. This is because the quantitative uncertainty measures can be propagated to the subsequent units, such as decision-making modules, to lead to safe maneuver planning or emergency braking, which is of utmost importance in safety-critical systems. Therefore, semantic segmentation predictions integrated with reliable confidence estimates can significantly reinforce the concept of safe autonomy. In this talk, I will introduce our recent neural network architecture, named SalsaNext, which can achieve uncertainty-aware semantic segmentation of full 3D LiDAR point clouds in real-time. Before diving into the SalsaNext model's technical details, I will give a gentle introduction to Bayesian deep learning and elaborate on different sources of uncertainties. Finally, I will present various quantitative and qualitative experiments on the large-scale challenging Semantic-KITTI dataset showing that SalsaNext significantly outperforms other state-of-the-art networks in terms of pixel-wise segmentation accuracy while having much fewer parameters, thus requiring less computation time. |
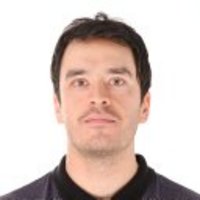
Speaker | Ekim Yurtsever |
Affiliation | The Ohio State University, Department of Electrical and Computer Engineering |
Title of the talk | Assessing Risk in Driving Videos using Deep Learning |
Abstract | Recently, increased public interest and market potential precipitated the emergence of self-driving platforms with varying degrees of automation. However, robust, fully automated driving in urban scenes is not achieved yet. In this talk, I will introduce a new concept called ‘end-to-end holistic driving risk perception’ with deep learning to alleviate the shortcomings of conventional risk assessment approaches. Holistic risk perception can be summarized as inferring the risk level of the driving scene with a direct mapping from the sensory input space. The proposed method applies semantic segmentation to individual video frames with a pre-trained model. Then, frames overlayed with these masks are fed into a time distributed CNN-LSTM network with a final softmax classification layer. This network was trained on a semi-naturalistic driving dataset with annotated risk labels. A comprehensive comparison of state-of-the-art pre-trained feature extractors was carried out to find the best network layout and training strategy. The best result, with a 0.937 AUC score, was obtained with the proposed framework. The code and trained models are available open-source. |
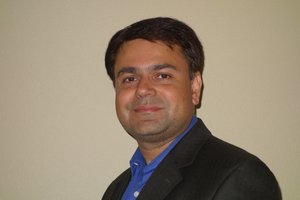
Speaker | Omesh Tickoo |
Affiliation | Intel Labs |
Title of the talk | Robust Visual Scene Understanding under Uncertainty |
Abstract | Deep Learning based perception systems have achieved high accuracy levels making them very attractive to be used for scene understanding applications. While most of the research and development effort in the area till date has focused on improving the accuracy rates, the black box nature of deep learning models is proving to be a hurdle for practical deployment in areas requiring robust and explainable solutions. In this talk we will present how acknowledging uncertainty and handling it in a principled manner in practical scenarios is essential to build robust AI models. The talk will outline our research on quantifying uncertainty to build robust and reliable scene understanding solutions that can adapt to varying scene conditions through novelty detection and active learning. |
Call For Papers
Spatio-temporal data is crucial to improve accuracy in deep learning applications. In this workshop, we mainly focus on data and deep learning, since data enables through applications to infer more information about environment for autonomous driving. This workshop will provide an opportunity to discuss applications and their data-dependent demands for understanding the environment of a vehicle while addressing how the data can be exploited to improve results instead of changing proposed architectures. The ambition of this full-day DDIVA workshop is to form a platform for exchanging ideas and linking the scientific community active in intelligent vehicles domain.
To this end we welcome contributions with a strong focus on (but not limited to) the following topics within Data Driven Intelligent Vehicle Applications:
Data Perspective:
- Synthetic Data Generation
- Sensor Data Synchronization
- Sequential Data Processing
- Data Labeling
- Data Visualization
- Data Discovery
Application Perspective:
- Visual Scene Understanding
- Large Scale Scene Reconstruction
- Semantic Segmentation
- Object Detection
- In Cabin Understanding
- Emotion Recognition
Contact workshop organizers: emec.ercelik( at )tum.de / burcu.karadeniz( at )in.tum.de
Submission
Authors are encouraged to submit high-quality, original (i.e. not been previously published or accepted for publication in substantially similar form in any peer-reviewed venue including journal, conference or workshop) research. Authors of accepted workshop papers will have their paper published in the conference proceeding. For publication, at least one author needs to be registered for the workshop and the conference and present their work.
While preparing your manuscript, please follow the formatting guidelines of IEEE available here and listed below. Papers submitted to this workshop as well as IV2020 must be original, not previously published or accepted for publication elsewhere, and they must not be submitted to any other event or publication during the entire review process.
Manuscript Guidelines:
- Language: English
- Paper size: US Letter
- Paper format: Two-column format in the IEEE style
- Paper limit: For the initial submission, a manuscript can be 6-8 pages. For the final submission, a manuscript should be 6 pages, with 2 additional pages allowed, but at an extra charge ($100 per page)
- Abstract limit: 300 words
- File format: A single PDF file, please limit the size of PDF to be 10 MB
- Compliance: check here for more info
The paper template is also identical to the main IV2020 symposium:
To go paper submission site, please click here.