Neuroscience and Motor Control
For understanding the human machine, understanding the guiding principles behind human motor control and formulate computational hypotheses about movement and interaction produced by the central nervous system is fundamental. Both, its building plan and the according control and learning system are tightly coupled with each other and give humans their incredible abilities to learn how to grasp and manipulate the world it. For understanding the computational principles behind one needs to ask which representations do so elegantly link the cognitive to the motor control level?
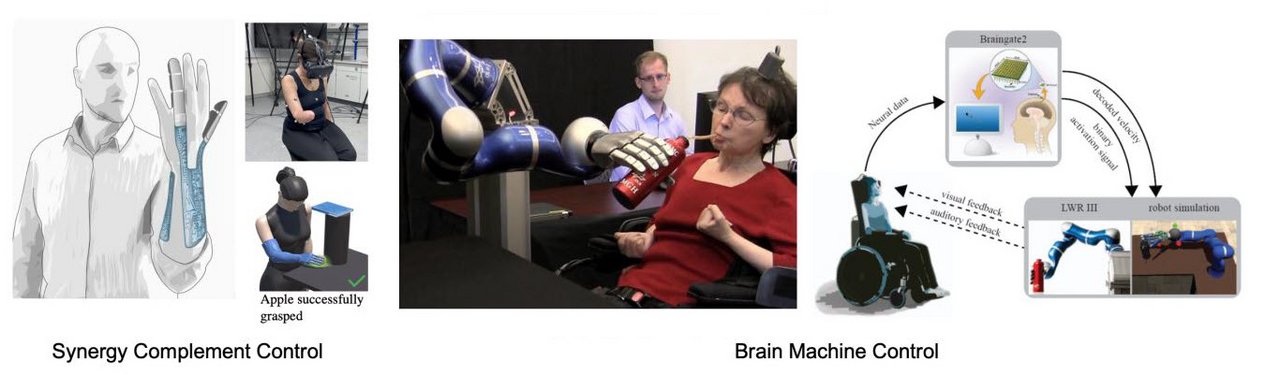
Contributions
From analyzing human collision pain tolerances and neuromechanical reflexes patterns [123] basis of human low-level controls for motion and redundancy resolution could be understood. Together with experts from human motor control we developed novel bio-inspired adaptive impedance control and learning algorithms [124] based on the systemic understanding of controllable muscle viscoelasticity and learned stretch reflexes [125]. The interpretation of the intrinsic muscle/tendon elasticities in humans could be extended by elaborating various model-based optimal control schemes for viscoelastic systems and varying compliance topology. By suitable interpretation of the found controls, underlying principles of the human musculoskeletal anatomy [126] could be better understood. Besides, reflex control models are studied [123] to introduce a new protective reflex methodology for robots and prostheses inspired by the analysis of human finger and arm retraction reflexes denoted artificial robot nervous system (aRNS) [75]. In addition, human-arm coordination is used in gripping tasks to develop a new control method for prostheses called synergy complement control. It naturally complements the user’s stump movements and adapts to new tasks online [128].
Regarding symbiotic control, we pioneered transferring mechatronics, robotics, and control theory into assistance systems [129]. Our first contribution was the successful continuous control of an entire hand-arm system with 19 degrees of freedom via invasive neural signals of a tetraplegic person [130, 131, 132, 133]. Following a simulative test phase lasting several years, a semi-autonomous control and planning system was designed that covers neural control, context sensitive task and reflex planning and safe interaction control. Furthermore, inspired by the idea of human upper limb synergies [134], we introduced the Synergy Complement Control (SCC) framework [128]. It enables the prosthesis to instantaneously complement the desired movement of a human residual limb autonomously and in accordance with human arm synergies.
In the field of multi-sensory human observation, we proposed digital twins models to archive the further benefit of improving our understanding of biological systems and have the potential to help us improve diagnosis, monitoring, and intervention in healthcare [68, 135, 136]. For this reason, we aim to develop both forward and inverse dynamic models, which may be run in real-time and, in combination with appropriate sensors, be used to monitor the human body [62, 63].
[62] Jumana Ma’touq, Tingli Hu, and Sami Haddadin. “A validated combined musculotendon path and muscle-joint kinematics model for the human hand”. In: Computer Methods in Biomechanics and Biomedical Engineering 22.7 (2019). PMID: 30880463, pp. 727–739.
[63] Tingli Hu, Johannes Kühn, and Sami Haddadin. “Forward and inverse dynamics modeling of human shoulder-arm musculoskeletal system with scapulothoracic constraint”. In: Computer Methods in Biomechanics and Biomedical Engineering 23.11 (2020). PMID: 32552013, pp. 785–803.
[68] Kim K. Peper, Dinmukhamed Zardykhan, Marion Egger, Martina Steinböck, Friedemann Müller, Xavier Hildenbrand, Alexander Koenig, Elisabeth R. Jensen, and Sami Haddadin. “Testing RobotBased Assist-as-Needed Therapy for Improving Active Participation of a Patient during Early Neurorehabilitation: A Case Study”. In: 2022 International Conference on Rehabilitation Robotics (ICORR). Rotterdam, Netherlands: IEEE Press, 2022, pp. 1–6.
[75] Johannes Kuehn and Sami Haddadin. “An Artificial Robot Nervous System To Teach Robots How To Feel Pain And Reflexively React To Potentially Damaging Contacts”. In: IEEE Robotics and Automation Letters 2.1 (Jan. 2017), pp. 72–79.
[123] Johannes Kuehn, Carlo Bagnato, Etienne Burdet, and Sami Haddadin. “Arm movement adaptation to concurrent pain constraints”. In: Scientific Reports 11 (2021).
[124] Chenguang Yang, Gowrishankar Ganesh, Sami Haddadin, Sven Parusel, Alin Albu-Schäffer, and Etienne Burdet. “Human-like adaptation of force and impedance in stable and unstable interactions”. In: IEEE Transactions on Robotics 27.5 (2011), pp. 918–930.
[125] Tingli Hu, Johannes Kühn, Jumana Ma’touq, and Sami Haddadin. “Learning and Identification of human upper-limb muscle synergies in daily-life tasks with autoencoders”. In: OTWorld Congress. Leipzig, Germany, May 2018.
[126] Diego Hidalgo-Carvajal, Christopher Herneth, Abdeldjallil Naceri, and Sami Haddadin. “End to-End From Human Hand Synergies to Robot Hand Tendon Routing”. In: IEEE Robotics and Automation Letters 7.4 (2022), pp. 10057–10064.
[128] Johannes Kühn. “Human-Inspired Reflexes and Coordination in Soft Robot and Upper-Limb Prosthesis Control”. Dissertation. München: Technische Universität München, 2022.
[129] Thomas Dickmann, Nikolas J. Wilhelm, Claudio Glowalla, Sami Haddadin, Patrick van der Smagt, and Rainer Burgkart. “An Adaptive Mechatronic Exoskeleton for Force-Controlled Finger Rehabilitation”. In: Frontiers in Robotics and AI 8 (2021).
[130] Jörn Vogel, Sami Haddadin, John D. Simeral, Sergey D. Stavisky, Dirk Bacher, Leigh R. Hochberg, John P. Donoghue, and Patrick van der Smagt. “Continuous Control of the DLR Light-WeightRobot III by a human with tetraplegia using theBrainGate2 Neural Interface System”. In: International Symposium on Experimental Robotics (ISER 2010). 2010.
[131] Leigh R. Hochberg, Daniel Bacher, Beata Jarosiewicz, Nicolas Y. Masse, John D. Simeral, Joern Vogel, Sami Haddadin, Jie Liu, Sydney S. Cash, Patrick van der Smagt, et al. “Reach and grasp by people with tetraplegia using a neurally controlled robotic arm”. In: Nature 485.7398 (2012), pp. 372–375.
[132] Jörn Vogel, Sami Haddadin, John D. Simeral, Sergey D. Stavisky, Daniel Bacher, Leigh R. Hochberg, John P. Donoghue, and Patrick van der Smagt. “Continuous Control of the DLR Light-Weight Robot III by a Human with Tetraplegia Using the BrainGate2 Neural Interface System”. In: Experimental Robotics. Ed. by Oussama Khatib, Vijay Kumar, and Gaurav Sukhatme. Vol. 79. Springer Tracts in Advanced Robotics. Berlin Heidelberg: Springer, 2014, pp. 125–136.
[133] Jörn Vogel, Sami Haddadin, Beata Jarosiewicz, John D. Simeral, Daniel Bacher, Leigh R. Hochberg, John P. Donoghue, and Patrick van der Smagt. “An assistive decision-and-control architecture for force-sensitive hand-arm systems driven by human-machine interfaces”. In: The International Journal of Robotics Research 34.6 (2015), pp. 763–780.
[134] Tingli Hu, Johannes Kuehn, and Sami Haddadin. “Identification of Human Shoulder-Arm Kinematic and Muscular Synergies During Daily-Life Manipulation Tasks”. In: 2018 7th IEEE International Conference on Biomedical Robotics and Biomechatronics (Biorob). 2018, pp. 1011– 1018.
[135] Mario Tröbinger, Andrei Costinescu, Hao Xing, Jean Elsner, Tingli Hu, Abdeldjallil Naceri, Luis Figueredo, Elisabeth Jensen, Darius Burschka, and Sami Haddadin. “A Dual Doctor-Patient Twin Paradigm for Transparent Remote Examination, Diagnosis, and Rehabilitation”. In: IEEE/RSJ International Conference on Intelligent Robots and Systems (IROS). 2021, pp. 2933–2940.
[136] Mario Tröbinger, Andrei Costinescu, Hao Xing, Jean Elsner, Tingli Hu, Abdeldjallil Naceri, Luis Figueredo, Elisabeth Jensen, Darius Burschka, and Sami Haddadin. “A Dual Doctor-Patient Twin Paradigm for Transparent Remote Examination, Diagnosis, and Rehabilitation”. In: 2021 IEEE/RSJ International Conference on Intelligent Robots and Systems (IROS). 2021, pp. 2933– 2940.