5th International Workshop on "Data-Driven Intelligent Vehicle Applications"
Introduction
This workshop aims to address the challenges in autonomous driving by focusing on Data and Application domains. Well-labeled data is crucial to improve accuracy in deep learning applications. In this workshop, we mainly focus on data and deep learning, since data enables through applications to infer more information about the environment for autonomous driving.
Recent advancements in processing units have improved our ability to construct a variety of architectures for understanding the surroundings of vehicles. Deep learning methods have been developed for geometric and semantic understanding of environments in driving scenarios aiming to increase the success of full autonomy with the cost of large amounts of data. Recently proposed methods challenge this dependency by pre-processing the data, enhancing, collecting, and labeling it intelligently. In addition, the dependency on data can be relieved by generating synthetic data, which alleviates this need with cost-free annotations. This workshop aims to form a platform for exchanging ideas and linking the scientific community active in the intelligent vehicles domain. This workshop will provide an opportunity to discuss applications and their data-dependent demands for the spatiotemporal understanding of the surroundings while addressing how the data can be exploited to improve results instead of changing proposed architectures.
Please click to view the workshop in the previous years.
Important Dates
DDIVA Workshop: June 2nd 2024
Please also check the conference web page for updates.
February 01, 2024: Workshop Paper Submission Deadline
March 30, 2024: Workshop Paper Notification of Acceptance
April 22, 2024: Workshop Final Paper Submission Deadline
Workshop Program
This workshop starts at 9:00 AM (local time).
Start | End | Program | Speaker | Title |
---|---|---|---|---|
08:00 | 09:00 | Registration | ||
09:00 | 09:10 | Opening & Welcome | Walter Zimmer (TUM) | |
09:10 | 09:25 | Keynote Presentation 1 | Prof. Dr. Mohan M. Trivedi (UCSD) | |
09:25 | 09:40 | Keynote Presentation 2 | Prof. Dr.-Ing. Christoph Stiller (KIT) | |
09:40 | 09:55 | Keynote Presentation 3 | Prof. Dr. Jiaqi Ma (UCLA) | |
09:55 | 10:10 | Keynote Presentation 4 | Dr. Ignazio Alvarez (Intel) | Challenges and Opportunities in Automated Driving in the era of Large Language Models |
10:10 | 10:25 | Keynote Presentation 5 | Tobias Fleck (FZI) | |
10:25 | 10:40 | Keynote Presentation 6 | Prof. Dr.-Ing. Andreas Festag (THI) | |
10:40 | 10:55 | Keynote Presentation 7 | Christian Geller (RWTH) | |
10:55 | 11:10 | Panel Discussion | ||
11:10 | 11:25 | Paper Presentation 1 | Esteban Rivera (TUM) | Camera-LiDAR Inconsistency Analysis for Active Learning in Object Detection |
11:25 | 11:40 | Paper Presentation 2 | Lars Ulrich (FAU) | Transfer Learning Study of Motion Transformer-based Trajectory Predictions |
11:40 | 11:55 | Paper Presentation 3 | Ross Greer (UCSD) | Learning to Find Missing Video Frames with Synthetic Data Augmentation: A General Framework and Application in Generating Thermal Images Using RGB Cameras |
11:55 | 12:00 | Closing | Walter Zimmer (TUM) |
Confirmed Paper Presentations

Speaker | Esteban Rivera, M.Sc. |
Affiliation | Technical University of Munich (TUM)
|
Title of the talk | Camera-LiDAR Inconsistency Analysis for Active Learning in Object Detection |
Abstract | Today, deep learning detectors for autonomous driving are delivering impressive results on public datasets and in real-world applications. However, these detectors require large amounts of data, especially labeled data, to achieve the performance needed to ensure safe driving. The process of collecting and tagging data is expensive and cumbersome. Therefore, the recent focus of the industry has been on how to achieve similar performance while limiting the amount of labeled data required to train such models. Within the cross-modal active learning paradigm, we propose and analyze new strategies to exploit the inconsistencies between camera and LiDAR detectors to improve sampling efficiency and label only the samples that bring the most improvement to model training. For this, we leverage the 2D projection of the bounding boxes to equalize the output quality of camera and LiDAR detections. Finally, we achieve up to 0.6% AP improvement for camera and 2% improvement for LiDAR over random sampling on the KITTI dataset using a sampling strategy based on the number of detected boxes. |

Speaker | Lars Ullrich, M.Sc. |
Affiliation | University of Erlangen–Nuremberg
|
Title of the talk | Transfer Learning Study of Motion Transformer-based Trajectory Predictions |
Abstract | Trajectory planning in autonomous driving is highly dependent on predicting the emergent behavior of other road users. Learning-based methods are currently showing impressive results in simulation-based challenges, with transformer-based architectures technologically leading the way. Ultimately, however, predictions are needed in the real world. In addition to the shifts from simulation to the real world, many vehicle- and country-specific shifts, i.e. differences in sensor systems, fusion and perception algorithms as well as traffic rules and laws, are on the agenda. Since models that can cover all system setups and design domains at once are not yet foreseeable, model adaptation plays a central role. Therefore, a simulation-based study on transfer learning techniques is conducted on basis of a transformer-based model. Furthermore, the study aims to provide insights into possible trade-offs between computational time and performance to support effective transfers into the real world. |

Speaker | Dr. Ross Greer |
Affiliation | University of California San Diego (UCSD)
|
Title of the talk | Learning to Find Missing Video Frames with Synthetic Data Augmentation: A General Framework and Application in Generating Thermal Images Using RGB Cameras |
Abstract | Advanced Driver Assistance Systems (ADAS) in intelligent vehicles rely on accurate driver perception within the vehicle cabin, often leveraging a combination of sensing modalities. However, these modalities operate at varying rates, posing challenges for real-time, comprehensive driver state monitoring. This paper addresses the issue of missing data due to sensor frame rate mismatches, introducing a generative model approach to create synthetic yet realistic thermal imagery. We propose using conditional generative adversarial networks (cGANs), specifically comparing the pix2pix and CycleGAN architectures. Experimental results demonstrate that pix2pix outperforms CycleGAN, and utilizing multi-view input styles, especially stacked views, enhances the accuracy of thermal image generation. Moreover, the study evaluates the model's generalizability across different subjects, revealing the importance of individualized training for optimal performance. The findings suggest the potential of generative models in addressing missing frames, advancing driver state monitoring for intelligent vehicles, and underscoring the need for continued research in model generalization and customization. |
Confirmed Keynote Speakers

Speaker | Prof. Dr. Mohan Trivedi |
Affiliation | University of California San Diego (UCSD) |
Title of the talk | Past, Present and Future in Safe Autonomous Driving: A Data-Centric Perspective |
Abstract: | The presentation is an attempt to identify challenging issues that require serious efforts and careful resolution to assure the safety, reliability and robustness of autonomous vehicles for operation on public roads. We will discuss issues related to the safe autonomous driving in the real-world environment, and cover important accomplishments of the field—particularly those enabled by advancements in computer vision, and machine learning-based human-centric approaches. We will highlight the role of both ‘knowledge-based” and “data-driven” approaches which enable novel, efficient, and practical driver assistance and highly autonomous automobiles. We will discuss how novel naturalistic driving studies support development of curated datasets, metrics and algorithms for situational criticality assessment, in-cabin activity monitoring, driver readiness, take-over time predication, predict intentions of surrounding agents, and plan/execute actions for safe & smooth maneuvers and control transitions. Links to Related Papers: http://cvrr.ucsd.edu/publications/index.html |
Bio | Mohan Trivedi is a Distinguished Professor of Engineering and founding director of the Computer Vision and Robotics Research Laboratory est. 1986) and the Laboratory for Intelligent and Safe Automobiles (LISA, est. 2001) at the University of California San Diego. LISA has played significant roles in the development of human-centered safe autonomous driving, advanced driver assistance systems, vision systems for intelligent transportation, homeland security, assistive technologies and human-robot interaction fields. He has mentored over 35 PhDs, 100 Masters and over 80 international research scholars. The LISA team has won over 30 “Best/Finalist” paper awards, six best dissertation awards and has received the IEEE Intelligent Transportation Systems (ITS) Society’s Outstanding Researcher Award and LEAD Institution Award, as well as the Meritorious Service Award of the IEEE Computer Society. Trivedi is a Life Fellow of IEEE, SPIE, IAPR a Distinguished Lecturer for the IEEE Robotics & Automation Society. Trivedi regularly serves as a consultant to various industry and government agencies in the US and abroad and serves on panels dealing with technological, strategic, privacy, and ethical issues. |

Speaker | Prof. Dr.-Ing. Christoph Stiller and Frank Bieder |
Affiliation | Karlsruhe Institute of Technology (KIT) |
Title of the talk | A data driven approach to scale automatic driving: Closing the cycle of semantic mapping, map verification and map perception |
Abstract: | |
Bio | Christoph Stiller studied Electrical Engineering (Dipl.-Ing.) in Aachen, Germany and Trondheim, Norway. After completion of his Dr.-Ing. degree (Ph.D.) in 1994 he spent a PostDoc year at INRS in Montreal, Canada. In 1995 he joined Robert Bosch GmbH. In 2001 he became chaired professor at Karlsruhe Institute of Technology, Germany. In 2010 he spent three months by invitation at CSIRO in Brisbane, Australia. In 2015 he spent a four month sabbatical with Bosch RTC and Stanford University in California. Dr. Stiller served as President of the IEEE Intelligent Transportation Systems Society (2012-2013) and was a Vice President before since 2006. He served as Editor-in-Chief of the IEEE Intelligent Transportation Systems Magazine (2009-2011) and as Associate Editor for several IEEE Transactions. He is recipient of the 2016 IEEE ITS Outstanding Application Award. |

Speaker | Prof. Dr. Jiaqi Ma |
Affiliation | University of California Los Angeles (UCLA) |
Title of the talk | |
Abstract: | |
Bio | Professor Jiaqi Ma is an Associate Professor at the UCLA Samueli School of Engineering and Associate Director of UCLA Institute of Transportation Studies. Prior to that, he was Assistant/Associate Professor and Academic Director of the University of Cincinnati Advanced Transportation Collaborative, Project Manager and Research Scientist with Leidos working at the Federal Highway Administration Turner-Fairbank Highway Research Center, and a contractor researcher at the Virginia Transportation Research Council of the Virginia Department of Transportation (DOT). He has led and managed many research projects worth of a total value of more than $20 million funded by U.S. DOT, NSF, state DOTs, and other federal/state/local programs covering areas of smart transportation systems, such as vehicle-highway automation, Intelligent Transportation Systems (ITS), connected vehicles, shared mobility, and large-scale smart system modeling and simulation, and artificial intelligence and advanced computing applications in transportation. He is Editor in Chief of the IEEE Open Journal of Intelligent Transportation Systems, and Associate Editor of Nature Scientific Reports, Journal of Intelligent Transportation Systems, and ASCE Open. He is Member of the Transportation Research Board (TRB) Standing Committee on Vehicle-Highway Automation, Member of TRB Standing Committee on Artificial Intelligence and Advanced Computing Applications, Member of American Society of Civil Engineers (ASCE) Connected & Autonomous Vehicles Impacts Committee, publication board member of IEEE ITS Society, Co-Chair of the IEEE ITS Society Technical Committee on Smart Mobility and Transportation 5.0. |

Speaker | Dr. Ignazio Alvarez |
Affiliation | Intel |
Title of the talk | Challenges and Opportunities in Automated Driving in the era of Large Language Models |
Abstract: | |
Bio | Ignacio Alvarez is a Senior Research Scientist at the Autonomous Driving Research Lab in Intel Labs where he develops, software, system architectures and simulation tools to accelerate the adoption of safe automated driving technologies. Previous to Intel, Ignacio worked for 8 years at BMW leading R&D and product development for Advanced Driver Assistance Systems and Vehicle Telematics Services Solutions in Europe, America and Asia. Ignacio received his International PhD in Computer Science from University of the Basque Country (Spain) and Clemson University (USA) in 2011, M.S. in Information Science in Offenburg University (Germany) and B.S. in Communication Science from Burgos University (Spain). Ignacio’s research is focused on the development of intelligent connected automated vehicles that augment human mobility with safer experiences. He has authored dozens of peer-reviewed papers, edited books on automated driving. |
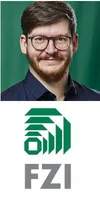
Speaker | Tobias Fleck |
Affiliation | FZI |
Title of the talk | |
Abstract: | |
Bio | Tobias Fleck studied computer science at the Karlsruhe Institute of Technology (KIT). During his master's degree, he worked on cognitive systems, with a particular focus on probabilistic estimation and modeling methods. He carried out his master's thesis "Trajectory Estimation and Prediction in the Context of Autonomous Driving" in the Technical Cognitive Systems (TKS) department. Since February 2017, Tobias Fleck has been working as a research assistant at the FZI Research Center for Computer Science in the TKS department. His research focuses on probabilistic, distributed sensor data fusion, multi-object tracking and machine learning. |

Speaker | Prof. Dr.-Ing. Andreas Festag |
Affiliation | Technical University Ingolstadt of Applied Sciences (THI) |
Title of the talk | |
Abstract: | |
Bio | Andreas Festag is a professor at Technische Hochschule Ingolstadt and with the research and test center for vehicle safety CARISSMA. He is also deputy head at the Fraunhofer Application Center »Connected Mobility and Infrastructure«. Andreas has worked on various research projects for wireless and mobile communication networks and published more than 100 papers in journals, conference proceedings and workshops. His research is concerned with architecture, design and performance evaluation of wireless and mobile communication systems and protocols, with a focus on vehicular communication and Intelligent Transportation Systems (ITS). He is senior member of IEEE. Andreas Festag received a diploma degree (1996) and Ph.D. (2003) in Electrical Engineering from the Technical University Berlin. As researcher, he worked with the Telecommunication Networks Group (TKN) at Technical University Berlin, Heinrich-Hertz-Institute (HHI) in Berlin, NEC Laboratories in Heidelberg, Vodafone chair Mobile Communication Systems at Technical University Dresden and Fraunhofer Institute for Transportation and Infrastructure Systems (IVI). |

Speaker | Apoorv Singh, M.Sc. |
Affiliation | Motional |
Title of the talk | Vision-based Perception for Autonomous Driving |
Abstract | The rapid development of autonomous vehicles has revolutionized the transportation industry, promising safer roads, reduced congestion, and increased accessibility. Central to the success of autonomous vehicles is their ability to perceive and interpret the surrounding environment accurately. This talk aims to explore the critical role of perception in autonomous vehicles and its impact on ensuring safe and efficient transportation. Safety is of paramount importance in autonomous driving, and perception plays a crucial role in identifying potential hazards and mitigating risks. The talk will address the strategies employed by Motional to tackle some of the SOTA techniques to tackle this hard problem. Additionally, the presentation will highlight the ongoing research and development efforts to enhance the perception systems' reliability, resilience, and adaptability. This talk will conclude by highlighting the future direction and potential advancements in perception technology, such as the integration of machine learning with smart data collection techniques, enabling autonomous vehicles to continually improve their perception capabilities. By attending this talk, participants will gain insights into the critical role of perception in autonomous vehicles, understand the challenges and advancements in perception technology, and appreciate its impact on achieving safe and efficient transportation in the era of autonomous driving. |
Bio | Apoorv Singh is a Tech Lead and Sr. Machine Learning researcher. He along with his team develops state-of-the-art computer vision systems to detect objects in real time for an online perception system. |
Call For Papers
This workshop aims to address the challenges in autonomous driving by focusing on Data and Application domains. Spatio-temporal data is crucial to improve accuracy in deep learning applications. In this workshop, we mainly focus on data and deep learning, since data enables through applications to infer more information about environment for autonomous driving. This workshop will provide an opportunity to discuss applications and their data-dependent demands for understanding the environment of a vehicle while addressing how the data can be exploited to improve results instead of changing proposed architectures. The ambition of this full-day DDIVA workshop is to form a platform for exchanging ideas and linking the scientific community active in intelligent vehicles domain.
To this end we welcome contributions with a strong focus on (but not limited to) the following topics within Data Driven Intelligent Vehicle Applications:
- Dataset Curation, Data Labeling
- 3D Object Detection
- Sensor Data Fusion
- Semantic Segmentation
- Sensor Calibration, Sensor Synchronization
- Active Learning, Uncertainty Quantification
- Cooperative Perception
- Multi-object Tracking
- Domain Adaptation
- Transfer Learning
- Traffic Simulation
- Sim2real Transfer
Contact workshop organizers: walter.zimmer( at )tum.de
Submission
Please check the conference webpage for the details of submission guidelines.
Authors are encouraged to submit high-quality, original (i.e. not been previously published or accepted for publication in substantially similar form in any peer-reviewed venue including journal, conference or workshop) research. Authors of accepted workshop papers will have their paper published in the conference proceeding. For publication, at least one author needs to be registered for the workshop and the conference and present their work.
While preparing your manuscript, please follow the formatting guidelines of IEEE available here and listed below. Papers submitted to this workshop as well as IV2024 must be original, not previously published or accepted for publication elsewhere, and they must not be submitted to any other event or publication during the entire review process.
Manuscript Guidelines:
- Language: English
- Paper size: US Letter
- Paper format: Two-column format in the IEEE style
- Paper limit: 6 pages, with max. 4 additional pages allowed, but at an extra charge ($100 per page)
- Abstract limit: 200 words
- File format: A single PDF file, please limit the size of PDF to be 10 MB
- Compliance: check here for more info
The paper template is also identical to the main IV2024 symposium:
Paper submission site: https://its.papercept.net/conferences/scripts/start.pl