4th International Workshop on "Data Driven Intelligent Vehicle Applications"
DDIVA 2023
June, 4th 2023 (8:00-17:00 PT, GMT-8)
A workshop in conjunction with IV 2023 in Anchorage, Alaska, USA
Registration via IV23 Poral Site. Please check the conference website for details.
IEEE ITSS members have free access to all workshops!
Apply here for IEEE ITSS membership.
Home
This workshop aims to address the challenges in autonomous driving by focusing on Data and Application domains. Well-labeled data is crucial to improve accuracy in deep learning applications. In this workshop, we mainly focus on data and deep learning, since data enables through applications to infer more information about the environment for autonomous driving.
Recent advancements in processing units have improved our ability to construct a variety of architectures for understanding the surroundings of vehicles. Deep learning methods have been developed for geometric and semantic understanding of environments in driving scenarios aiming to increase the success of full autonomy with the cost of large amounts of data. Recently proposed methods challenge this dependency by pre-processing the data, enhancing, collecting, and labeling it intelligently. In addition, the dependency on data can be relieved by generating synthetic data, which alleviates this need with cost-free annotations. This workshop aims to form a platform for exchanging ideas and linking the scientific community active in the intelligent vehicles domain. This workshop will provide an opportunity to discuss applications and their data-dependent demands for the spatiotemporal understanding of the surroundings while addressing how the data can be exploited to improve results instead of changing proposed architectures.
Please click to view the workshop in the previous years.
Important Dates
DDIVA Workshop: June 4th 2023
Please also check the conference web page for updates.
February 01, 2023: Workshop Paper Submission Deadline
March 30, 2023: Workshop Paper Notification of Acceptance
April 22, 2023: Workshop Final Paper Submission Deadline
Workshop Program

This workshop starts at 8:30 am AKDT (Alaska Daylight Time, GMT-8), local time in Anchorage.
Start | End | Program | Speaker | Title |
---|---|---|---|---|
08:00 | 08:30 | Registration | ||
08:30 | 08:40 | Opening & Welcome | ||
08:40 | 09:10 | Keynote Presentation 1 | Roland Meertens, M.Sc. | Data-centric AI for Robotics |
09:10 | 09:40 | Keynote Presentation 2 | Prof. Dr. Fatma Güney | Efficient Multi-Agent Trajectory Prediction and Unknown Object Segmentation |
09:40 | 10:10 | Keynote Presentation 3 | Dr.-Ing. Alexander Wischnewski | Data-driven development for perception software functions with application to autonomous commercial vehicles |
10:10 | 10:15 | Coffee Break | ||
10:15 | 10:45 | Keynote Presentation 4 | Prof. Dr. Abhinav Valada | Rethinking Learning for Autonomy at Scale |
10:45 | 11:15 | Keynote Presentation 5 | Dr.-Ing. Oliver Grau | The role of synthetic data in the development of ML-based perception modules |
11:15 | 11:45 | Keynote Presentation 6 | Prof. Julian Kooij (PhD) | Vehicle localization without HD Map: learning to find your camera’s pose in an aerial image |
11:45 | 12:15 | Keynote Presentation 7 | Andras Palffy (PhD) | Navigating Multi-Sensor Datasets: A Deep Dive into Radar Data |
12:15 | 12:40 | Panel Discussion 1 | ||
12:40 | 13:40 | Lunch Break | ||
13:40 | 14:10 | Keynote Presentation 8 | Prof. Dr.-Ing. Alina Roitberg | Data-driven driver observation: towards adaptable, data-efficient and uncertainty-aware models |
14:10 | 14:40 | Keynote Presentation 9 | Ekim Yurtsever (PhD) | Explainable Driving with Deep Reinforcement Learning & Hierarchical State Representation |
14:40 | 15:10 | Keynote Presentation 10 | Akshay Rangesh (PhD) | Leveraging Data to Model Unknowns |
15:10 | 15:25 | Coffee Break | ||
15:25 | 15:55 | Keynote Presentation 11 | Prof. Mohan Trivedi (PhD) | Past, Present and Future in Safe Autonomous Driving: A Data-Centric Perspective |
15:55 | 16:10 | Paper Presentation | Ross Greer, M.Sc. | Pedestrian Advisories for Enhanced Safety Using Behavior Maps: Computational Framework and Experimental Analysis With Real-World Data |
16:10 | 16:40 | Keynote Presentation 12 | Apoorv Singh, M.Sc. | Building Scalable Perception Systems for Autonomous Vehicles Using Machine Learning |
16:40 | 17:10 | Keynote Presentation 13 | Philipp Karle, M.Sc. | Multi-Modal Sensor Fusion and Object Tracking for Autonomous Racing |
17:10 | 17:40 | Panel Discussion 2 | ||
17:40 | 17:55 | Closing |
Confirmed Paper Presentations
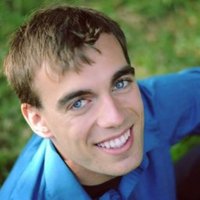

Speaker | Ross Greer, M.Sc. |
Affiliation | University of California San Diego (UCSD)
|
Title of the talk | Pedestrian Advisories for Enhanced Safety Using Behavior Maps: Computational Framework and Experimental Analysis With Real-World Data |
Abstract | It is critical for vehicles to prevent any collisions with pedestrians. Current methods for pedestrian collision prevention focus on integrating visual pedestrian detectors with Automatic Emergency Braking (AEB) systems which can trigger warnings and apply brakes as a pedestrian enters a vehicle’s path. Unfortunately, pedestrian-detection-based systems can be hindered in certain situations such as nighttime or when pedestrians are occluded. Our system addresses such issues using an online, map-based pedestrian detection aggregation system where common pedestrian locations are learned after repeated passes of locations. Using a carefully collected and annotated dataset in La Jolla, CA, we demonstrate the system’s ability to learn pedestrian zones and generate advisory notices when a vehicle is approaching a pedestrian despite challenges like dark lighting or pedestrian occlusion. Using the number of correct advisories, false advisories, and missed advisories to define precision and recall performance metrics, we evaluate our system and discuss future positive effects with further data collection. |
Confirmed Keynote Speakers
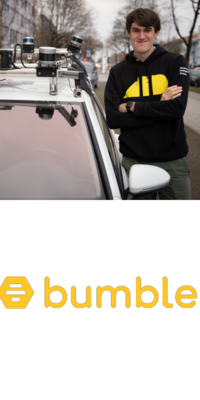
Speaker | Roland Meertens, M.Sc. |
Affiliation | Bumble |
Title of the talk | Data-centric AI for Robotics |
Abstract | After many years of advancements in convolutional neural networks we now see many interesting applications emerge from so-called foundational models. In this presentation we will discuss what models exist, what they can do, and how you can use these models. We will also cover some basic techniques of data selection which I believe companies and academia should put more focus on. |
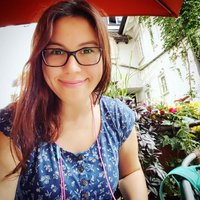

Speaker | Prof. Dr. Fatma Guney |
Affiliation | Dept. of Computer Engineering at Koç University in Istanbul |
Title of the talk | Efficient Multi-Agent Trajectory Prediction and Unknown Object Segmentation |
Abstract | In the first part, we will assume perfect perception information and talk about forecasting the future trajectories of agents in complex traffic scenes. While safe navigation requires reliable and efficient predictions for all agents in the scene, the standard paradigm is simply predicting the trajectory of a single agent of interest by orienting the scene according to the agent. The straightforward extensions to multi-agent predictions result in inefficient solutions that require iterating over each agent. We propose ADAPT, a novel approach for jointly predicting the trajectories of all agents in the scene with dynamic weight learning. We demonstrate that the prediction head can adapt to each agent's situation efficiently. ADAPT outperforms the state-of-the-art methods at a fraction of their computational overhead on both single-agent setting of the Argoverse and multi-agent setting of the Interaction. Our analyses show that ADAPT can focus on each agent with adaptive prediction without sacrificing efficiency. In the second part, we will break that assumption and focus on unknown object segmentation. Semantic segmentation methods typically perform per-pixel classification by assuming a fixed set of semantic categories. While they perform well on the known set, the network fails to learn the concept of objectness, which is necessary for identifying unknown objects. We explore the potential of query-based mask classification for unknown object segmentation. We discover that object queries specialize in predicting a certain class and behave like one vs. all classifiers, allowing us to detect unknowns by finding regions that are ignored by all the queries. Based on a detailed analysis of the model's behavior, we propose a novel anomaly scoring function. We demonstrate that mask classification helps to preserve the objectness and the proposed scoring function eliminates irrelevant sources of uncertainty. Our method achieves consistent improvements in multiple benchmarks, even under high domain shift, without retraining or using outlier data. With modest supervision for outliers, we show that further improvements can be achieved without affecting the closed-set performance. |


Speaker | Dr.-Ing. Oliver Grau |
Affiliation | Intel Labs |
Title of the talk | The role of synthetic data in the development of ML-based perception modules |
Abstract | Perception is the central component of any automated system. With the introduction of machine learning (ML)-trained deep neural networks (DNNs) as core technology of perception modules, the importance of data for training, but also validation is evident. This presentation contributes to the question of how synthetic data can be used in the AI development process, to improve quality and performance of DNNs and to detect flaws and weaknesses in the validation process. We present VALERIE, our a fully automated parametric synthetic data generation pipeline to generate complex and highly realistic (RGB) sensor data. The demonstrated target is in the domain of urban street scenes for the use in automated driving scenarios. Further, we describe how the metadata from this system can be used to improve the quality and understanding of training and validation datasets. |

Speaker | Prof. Dr. Abhinav Valada |
Affiliation | Robot Learning Lab, Albert-Ludwigs-Universität Freiburg |
Title of the talk | (Self-)Supervised Learning for Perception and Tracking in Autonomous Driving |
Abstract | Scene understanding and object tracking play a critical role in enabling autonomous vehicles to navigate in dense urban environments. The last decade has witnessed unprecedented progress in these tasks by exploiting learning techniques to improve performance and robustness. Despite these advances, most techniques today still require a tremendous amount of human-annotated training data and do not generalize well to diverse real-world environments. In this talk, I will discuss some of our efforts targeted at addressing these challenges by leveraging self-supervision and multi-task learning for various tasks ranging from panoptic segmentation to cross-modal object tracking using sound. Finally, I will conclude the talk with a discussion on opportunities for further scaling up the learning of these tasks. |
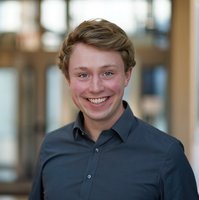

Speaker | Dr.-Ing. Alexander Wischnewski |
Affiliation | Driveblocks GmbH |
Title of the talk | Data-driven development for perception software functions with application to autonomous commercial vehicles |
Abstract | Alexander Wischnewski was awarded a B.Eng. in Mechatronics by the DHBW Stuttgart in 2015, and an M.Sc. in Electrical Engineering and Information Technology by the University of Duisburg-Essen, Germany, in 2017. He did research on autonomous vehicle motion control at the Chair of Automatic Control at TUM from 2017 until 2022 and led the TUM Autonomous Motorsport to a successful entry in the Indy Autonomous Challenge. He is now co-founder and CTO of driveblocks, an autonomous driving software startup based in Munich. |


Speaker | Prof. Julian Kooij (PhD) |
Affiliation | Intelligent Vehicles Group, TU Delft |
Title of the talk | Vehicle localization without HD Map: learning to find your camera’s pose in an aerial image |
Abstract | This work addresses cross-view camera pose estimation, i.e., determining the 3-Degrees-of-Freedom camera pose of a given ground-level image w.r.t. an aerial image of the local area. We propose SliceMatch, which consists of ground and aerial feature extractors, feature aggregators, and a pose predictor. The feature extractors extract dense features from the ground and aerial images. Given a set of candidate camera poses, the feature aggregators construct a single ground descriptor and a set of pose-dependent aerial descriptors. Notably, our novel aerial feature aggregator has a cross-view attention module for ground-view guided aerial feature selection and utilizes the geometric projection of the ground camera's viewing frustum on the aerial image to pool features. The efficient construction of aerial descriptors is achieved using precomputed masks. SliceMatch is trained using contrastive learning and pose estimation is formulated as a similarity comparison between the ground descriptor and the aerial descriptors. Compared to the state-of-the-art, SliceMatch achieves a 19% lower median localization error on the VIGOR benchmark using the same VGG16 backbone at 150 frames per second, and a 50% lower error when using a ResNet50 backbone. |

Speaker | Andras Palffy (PhD) |
Affiliation | Technical University of Delft & Perciv AI |
Title of the talk | Navigating Multi-Sensor Datasets: A Deep Dive into Radar Data |
Abstract | In my talk, I'll explore the important role of automotive radars, discussing the benefits of their use and the challenges we may face during data recording, management, annotation, and fusion. I will present our latest "View-of-Delft" dataset as a case study, demonstrating the surprising effectiveness of these sensors, while also acknowledging their limitations. To conclude, we'll examine how unlabeled data can be used for radar-related tasks, drawing from three practical examples of cross-sensor annotation/supervision. |
Bio | Andras Palffy received his MSc degree in 2016 in computer science Engineering from Pazmany Peter Catholic University, Hungary. He also obtained a MSc degree in Digital Signal and Image Processing from Cranfield University, United Kingdom, in 2015. From 2013 to 2017, he was employed by the startup Eutecus, developing computer vision algorithms for traffic monitoring and driver assistance applications. He received a Ph.D. degree at TU Delft, ME, Intelligent Vehicles group, focusing on radar based vulnerable road user detection for autonomous driving. Currently he is a postoc at TU Delft working on the same topic, but with an enhanced focus on low-level radar data representations. Last year, along with two co-founders he also started a startup named Perciv AI, a machine perception software company with a special focus on AI solutions for radars. |

Speaker | Prof. Dr.-Ing. Alina Roitberg |
Affiliation | Autonomous Sensing and Perception Lab, University of Stuttgart, Germany |
Title of the talk | Data-driven driver observation: towards adaptable, data-efficient and uncertainty-aware models |
Abstract | This talk will explore recent advances in video-based driver observation techniques aimed at creating adaptable, data-efficient, and uncertainty-aware solutions for in-vehicle monitoring. Topics covered will include: (1) an overview state-of-the-art methods and public datasets for driver activity analysis (2) the importance of adaptability in driver observation systems to cater to new situations (environments, vehicle types, driver behaviours) as well as strategies for addressing such open world tasks, and (3) incorporating uncertainty-aware approaches, vital for robust and safe decision-making. The talk will conclude with a discussion of future research directions and the potential applications of this technology, such as improving driver safety and improving the overall driving experience. |
Bio | Alina Roitberg is an Assistant Professor and leader of the newly established Autonomous Sensing and Perception Group at the University of Stuttgart. She received her B.Sc. and M.Sc. degrees in Computer Science from Technical University of Munich (TUM) in 2015. After working as a data science consultant in the automotive sector (2016-2017), she joined Karlsruhe Institute of Technology (KIT) for doctoral studies, during which she completed a Ph.D. internship at Facebook. She received her Ph.D. in deep learning for driver observation in 2021 and was subsequently a postdoctoral researcher at the Computer Vision for Human-Computer Interaction Lab at KIT. Her research interests include human activity recognition, uncertainty-aware deep learning, open-set, zero- and few-shot recognition. Her research was recognized with multiple awards, such as best student paper award at IV 2020 and best dissertation award by the German Chapter of the IEEE Intelligent Transportation Systems Society. |

Speaker | Ekim Yurtsever (PhD) |
Affiliation | The Ohio State University |
Title of the talk | Explainable Driving with Deep Reinforcement Learning & Hierarchical State Representation |
Abstract | End-to-end deep learning models are opaque. For safety-critical applications, interpretability is arguably as important as operational robustness. Here we propose a human-interpretable reinforcement learning-based automated driving agent. The system leverages a two-layer hierarchical structure. First, a high-level controller provides long-term interpretable driving goals. The second controller is trained to follow these commands while maintaining an optimal driving policy. Experimental results show that the proposed method can plan its path and navigate between randomly chosen origin-destination points in CARLA, a dynamic urban simulation environment. |
Bio | Ekim Yurtsever received his Ph.D. in Information Science in 2019 from Nagoya University, Japan. Since 2019, he has been with the Department of Electrical and Computer Engineering, The Ohio State University as a research associate. He also teaches image processing courses at undergraduate and graduate levels. His research interests include artificial intelligence, machine learning, computer vision, reinforcement learning, intelligent transportation systems, and automated driving systems. Currently, he is working on bridging the gap between the advances in the machine learning field and the intelligent vehicle domain. |
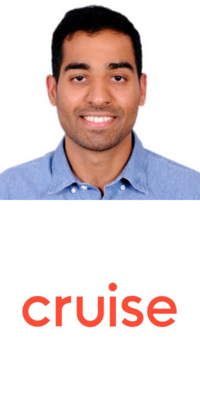
Speaker | Akshay Rangesh (PhD) |
Affiliation | Cruise |
Title of the talk | Leveraging Data to Model Unknowns |
Abstract | ML engineers at Cruise constantly leverage vast amounts of data produced by our sizable AV fleet to iteratively improve our models on a variety of tasks. This talk will cover one such task and demonstrate how we use auto-labelled datasets and continuous learning to improve AV behavior in perpetuity. In particular, I will talk about how we model the lifetime of unknown regions in space (i.e. occlusions), and discuss the entire process that is behind effectively solving such a task - from data mining, auto-labelling, model design and deployment, to continuous iteration and improvement. This talk will give a glimpse into the life cycle of AI models in commercial AVs, and present the framework needed to rapidly scale such AVs across multiple geographical regions and conditions. |
Bio | Akshay Rangesh is a Senior Applied Research Scientist at Cruise where he works for the Behaviors AI team. His research interests span computer vision and machine learning with a focus on object detection and tracking, human activity recognition, driver behavior analysis, and driver safety systems in general. He is also particularly interested in using sensor fusion and multi-modal approaches to create real time algorithms and models. Akshay received his Master’s and PhD in Electrical Engineering at UC San Diego where he was advised by Prof. Mohan M. Trivedi. During his time at the Laboratory for Intelligent & Safe Automobiles (LISA), he helped develop unique vehicular testbeds, models, and algorithms to enhance driver and vehicle safety. |
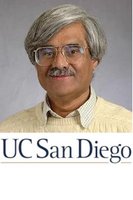

Speaker | Prof. Mohan Trivedi (PhD) |
Affiliation | University of California San Diego (UCSD) |
Title of the talk | Past, Present and Future in Safe Autonomous Driving: A Data-Centric Perspective |
Abstract: | The presentation is an attempt to identify challenging issues that require serious efforts and careful resolution to assure the safety, reliability and robustness of autonomous vehicles for operation on public roads. We will discuss issues related to the safe autonomous driving in the real-world environment, and cover important accomplishments of the field—particularly those enabled by advancements in computer vision, and machine learning-based human-centric approaches. We will highlight the role of both ‘knowledge-based” and “data-driven” approaches which enable novel, efficient, and practical driver assistance and highly autonomous automobiles. We will discuss how novel naturalistic driving studies support development of curated datasets, metrics and algorithms for situational criticality assessment, in-cabin activity monitoring, driver readiness, take-over time predication, predict intentions of surrounding agents, and plan/execute actions for safe & smooth maneuvers and control transitions. Links to Related Papers: http://cvrr.ucsd.edu/publications/index.html |
Bio | Mohan Trivedi is a Distinguished Professor of Engineering and founding director of the Computer Vision and Robotics Research Laboratory est. 1986) and the Laboratory for Intelligent and Safe Automobiles (LISA, est. 2001) at the University of California San Diego. LISA has played significant roles in the development of human-centered safe autonomous driving, advanced driver assistance systems, vision systems for intelligent transportation, homeland security, assistive technologies and human-robot interaction fields. He has mentored over 35 PhDs, 100 Masters and over 80 international research scholars. The LISA team has won over 30 “Best/Finalist” paper awards, six best dissertation awards and has received the IEEE Intelligent Transportation Systems (ITS) Society’s Outstanding Researcher Award and LEAD Institution Award, as well as the Meritorious Service Award of the IEEE Computer Society. Trivedi is a Life Fellow of IEEE, SPIE, IAPR a Distinguished Lecturer for the IEEE Robotics & Automation Society. Trivedi regularly serves as a consultant to various industry and government agencies in the US and abroad and serves on panels dealing with technological, strategic, privacy, and ethical issues. |

Speaker | Apoorv Singh, M.Sc. |
Affiliation | Motional |
Title of the talk | Building Scalable Perception Systems for Autonomous Vehicles Using Machine Learning |
Abstract | The rapid development of autonomous vehicles has revolutionized the transportation industry, promising safer roads, reduced congestion, and increased accessibility. Central to the success of autonomous vehicles is their ability to perceive and interpret the surrounding environment accurately. This talk aims to explore the critical role of perception in autonomous vehicles and its impact on ensuring safe and efficient transportation. Safety is of paramount importance in autonomous driving, and perception plays a crucial role in identifying potential hazards and mitigating risks. The talk will address the strategies employed by Motional to tackle some of the SOTA techniques to tackle this hard problem. Additionally, the presentation will highlight the ongoing research and development efforts to enhance the perception systems' reliability, resilience, and adaptability. This talk will conclude by highlighting the future direction and potential advancements in perception technology, such as the integration of machine learning with smart data collection techniques, enabling autonomous vehicles to continually improve their perception capabilities. By attending this talk, participants will gain insights into the critical role of perception in autonomous vehicles, understand the challenges and advancements in perception technology, and appreciate its impact on achieving safe and efficient transportation in the era of autonomous driving. |
Bio | Apoorv Singh is a Tech Lead and Sr. Machine Learning researcher. He along with his team develops state-of-the-art computer vision systems to detect objects in real time for an online perception system. |

Speaker | Phillip Karle, M.Sc. |
Affiliation | Technical University of Munich (TUM) |
Title of the talk | Multi-Modal Sensor Fusion and Object Tracking for Autonomous Racing |
Abstract | Reliable detection and tracking of surrounding objects are indispensable for comprehensive motion prediction and planning of autonomous vehicles. Due to the limitations of individual sensors, the fusion of multiple sensor modalities is required to improve the overall detection capabilities. Additionally, robust motion tracking is essential for reducing the effect of sensor noise and improving state estimation accuracy. The reliability of the autonomous vehicle software becomes even more relevant in complex, adversarial high-speed scenarios at the vehicle handling limits in autonomous racing. In this paper, we present a modular multi-modal sensor fusion and tracking method for high-speed applications. The method is based on the Extended Kalman Filter (EKF) and is capable of fusing heterogeneous detection inputs to track surrounding objects consistently. A novel delay compensation approach enables to reduce the influence of the perception software latency and to output an updated object list. It is the first fusion and tracking method validated in high-speed real-world scenarios at the Indy Autonomous Challenge 2021 and the Autonomous Challenge at CES (AC@CES) 2022, proving its robustness and computational efficiency on embedded systems. It does not require any labeled data and achieves position tracking residuals below 0.1 m. The related code is available as open-source software at github.com/TUMFTM/FusionTracking . |
Call For Papers
This workshop aims to address the challenges in autonomous driving by focusing on Data and Application domains. Spatio-temporal data is crucial to improve accuracy in deep learning applications. In this workshop, we mainly focus on data and deep learning, since data enables through applications to infer more information about environment for autonomous driving. This workshop will provide an opportunity to discuss applications and their data-dependent demands for understanding the environment of a vehicle while addressing how the data can be exploited to improve results instead of changing proposed architectures. The ambition of this full-day DDIVA workshop is to form a platform for exchanging ideas and linking the scientific community active in intelligent vehicles domain.
To this end we welcome contributions with a strong focus on (but not limited to) the following topics within Data Driven Intelligent Vehicle Applications:
Data Perspective:
- Synthetic Data Generation
- Sensor Calibration and Data Synchronization
- Data Pre-processing
- Data Labeling
- Active Learning
- Data Visualization
Application Perspective:
- Semantic Segmentation
- Object Detection and Tracking
- Point Cloud Processing
- Simulation
- Domain Adaptation
Contact workshop organizers: walter.zimmer( at )tum.de
Submission
Please check the conference webpage for the details of submission guidelines.
Authors are encouraged to submit high-quality, original (i.e. not been previously published or accepted for publication in substantially similar form in any peer-reviewed venue including journal, conference or workshop) research. Authors of accepted workshop papers will have their paper published in the conference proceeding. For publication, at least one author needs to be registered for the workshop and the conference and present their work.
While preparing your manuscript, please follow the formatting guidelines of IEEE available here and listed below. Papers submitted to this workshop as well as IV2023 must be original, not previously published or accepted for publication elsewhere, and they must not be submitted to any other event or publication during the entire review process.
Manuscript Guidelines:
- Language: English
- Paper size: US Letter
- Paper format: Two-column format in the IEEE style
- Paper limit: 6 pages, with max. 4 additional pages allowed, but at an extra charge ($100 per page)
- Abstract limit: 200 words
- File format: A single PDF file, please limit the size of PDF to be 10 MB
- Compliance: check here for more info
The paper template is also identical to the main IV2023 symposium:
Paper submission site: 2023.ieee-iv.org/workshops/