AI4DI
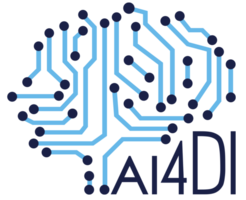
Artificial Intelligence for Digitalizing Industry
Mission
The mission of the AI4DI project is to make Europe the leader in Silicon-born Artificial Intelligence for accelerated edge processing. This means to bring the Artificial Intelligence from the cloud to the edge while making it resilient, safe, and secure for the manufacturing and process technologies of the future.
Objectives
The objective of the AI4DI project is to research and develop AI technologies implemented to different industrial sector applications and deployed under conditions as close as possible to real-life. The project aims to enhance processes based on repetitive tasks, focusing on replacing process identification and validation methods with intelligent technologies across industries such as automotive, semiconductor, machinery, food and beverage, and transportation.
AI4DI is building a roadmap on AI-based technologies, exploitation studies, business cases that are supporting accelerating the adoption of AI hardware (HW) and software (SW) solutions by the European industry for approaching its most urgent priorities in digitalisation and moving the intelligence to the edge and providing new distributed reference architectures that are aligned with the industrial requirements. Growing data factors cause the demand for high AI performance (e.g., data quality) and industrial advancements (e.g., moving from linear to network-based manufacturing processes). AI4DI is developing industry-grade AI technologies tailored to the European industry's specific needs based on various industrial sectors' requirements and specifications.
AI4DI provides a high-level reference hybrid system architecture for the digitalising industry and defines the different building blocks, functions, interactions, and workflows based on AI and IIoT solutions for seamless integration and scalability. The hybrid system architecture is applied to distributed and heterogeneous systems as reflected by the AI4DI applications in the different sectors.
The project's ultimate goal is to provide AI-based technologies at the edge for digitalising the industry by reducing costs, save time, optimising/improving processes/products/services, increasing quality by enhancing industrial processes, and built and sustain a dynamic AI technology ecosystem in Europe.
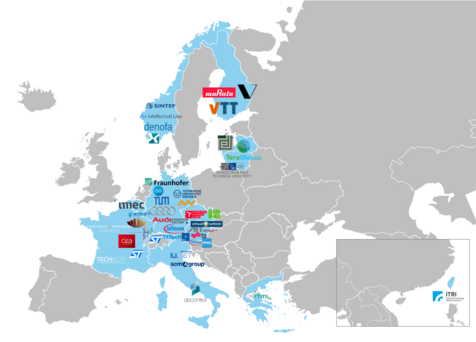
Funding
AI4DI has received funding within the Electronic Components and Systems for European Leadership Joint Undertaking (ECSEL JU) in collaboration with the European Union's H2020 Framework Programme and National Authorities, under grant agreement n° 826060.
The project consortium involves 38 partners across five supply chains.
To learn more about AI4DI, please refer to:
Project homepage
LinkedIn channel
Twitter channel
Research
Virtual AI Training Platform for Robot Learning
Current state-of-the-art approaches in Artificial Intelligence (AI) require large amounts of training data to solve industrial challenges. It is worth mentioning that the performance is highly dependent on the size and quality of the training data. While large datasets of real-world training data exist or can easily be generated for some problem domains, in robotics data is particularly hard to acquire due to the needed effort, time and hardware resources, or due to safety precautions.
To this end, the TUM team under the AI4DI project is developing simulation environments in which AI agents can be virtually-trained to control robots to execute industry-relevant challenges. To provide a high level of realism in simulated physics and rendering, we use high-end physics simulators and game development engines. In the video, we demonstrate how the virtual environment can be used to train an AI agent for solving a robotic manipulation task using reinforcement learning.
The benefits of fast and safe training in simulation come with the drawback of limited transferability of the simulation-trained AI agents to control real robots. This is still a major challenge due to the mismatch between the simulation and the real-world environment. In the next step of our research, we are working on approaches that can reduce the effects of the so-called reality gap and improve the transferability to real robots.